Deep mask estimation¶
Wang, Zhong-Qiu, Jonathan Le Roux, and John R. Hershey. “Alternative objective functions for deep clustering.” 2018 IEEE International Conference on Acoustics, Speech and Signal Processing (ICASSP). IEEE, 2018.
Yu, Dong, et al. “Permutation invariant training of deep models for speaker-independent multi-talker speech separation.” 2017 IEEE International Conference on Acoustics, Speech and Signal Processing (ICASSP). IEEE, 2017.
Kolbæk, Morten, et al. “Multitalker speech separation with utterance-level permutation invariant training of deep recurrent neural networks.” IEEE/ACM Transactions on Audio, Speech, and Language Processing 25.10 (2017): 1901-1913.
@inproceedings{wang2018alternative,
title={Alternative objective functions for deep clustering},
author={Wang, Zhong-Qiu and Le Roux, Jonathan and Hershey, John R},
booktitle={2018 IEEE International Conference on Acoustics, Speech and Signal Processing (ICASSP)},
pages={686--690},
year={2018},
organization={IEEE}
}
@inproceedings{yu2017permutation,
title={Permutation invariant training of deep models for speaker-independent multi-talker speech separation},
author={Yu, Dong and Kolb{\ae}k, Morten and Tan, Zheng-Hua and Jensen, Jesper},
booktitle={2017 IEEE International Conference on Acoustics, Speech and Signal Processing (ICASSP)},
pages={241--245},
year={2017},
organization={IEEE}
}
@article{kolbaek2017multitalker,
title={Multitalker speech separation with utterance-level permutation invariant training of deep recurrent neural networks},
author={Kolb{\ae}k, Morten and Yu, Dong and Tan, Zheng-Hua and Jensen, Jesper},
journal={IEEE/ACM Transactions on Audio, Speech, and Language Processing},
volume={25},
number={10},
pages={1901--1913},
year={2017},
publisher={IEEE}
}
[1]:
import nussl
import matplotlib.pyplot as plt
import time
import warnings
warnings.filterwarnings("ignore")
start_time = time.time()
def visualize_and_embed(sources):
plt.figure(figsize=(10, 6))
plt.subplot(211)
nussl.utils.visualize_sources_as_masks(sources,
y_axis='linear', db_cutoff=-40, alpha_amount=2.0)
plt.subplot(212)
nussl.utils.visualize_sources_as_waveform(
sources, show_legend=False)
plt.show()
nussl.play_utils.multitrack(sources)
model_path = nussl.efz_utils.download_trained_model(
'mask-inference-wsj2mix-model-v1.pth')
audio_path = nussl.efz_utils.download_audio_file(
'wsj_speech_mixture_ViCfBJj.mp3')
audio_signal = nussl.AudioSignal(audio_path)
separator = nussl.separation.deep.DeepMaskEstimation(
audio_signal, mask_type='soft', model_path=model_path)
estimates = separator()
estimates = {
f'Speaker {i}': e for i, e in enumerate(estimates)
}
visualize_and_embed(estimates)
Matching file found at /home/pseetharaman/.nussl/models/mask-inference-wsj2mix-model-v1.pth, skipping download.
Matching file found at /home/pseetharaman/.nussl/audio/wsj_speech_mixture_ViCfBJj.mp3, skipping download.
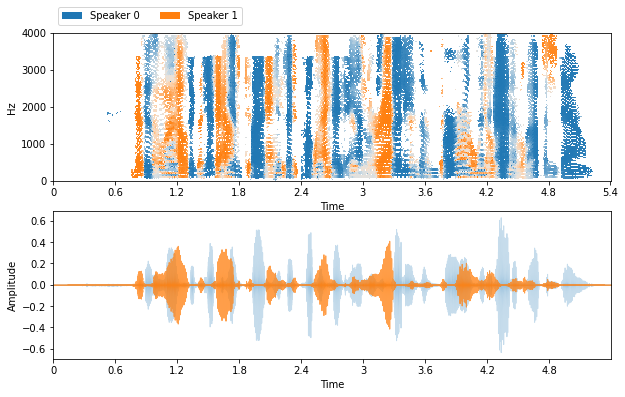
[2]:
end_time = time.time()
time_taken = end_time - start_time
print(f'Time taken: {time_taken:.4f} seconds')
Time taken: 5.5220 seconds